by Bogdan Djukic (Vay Co-Founder)
Introduction
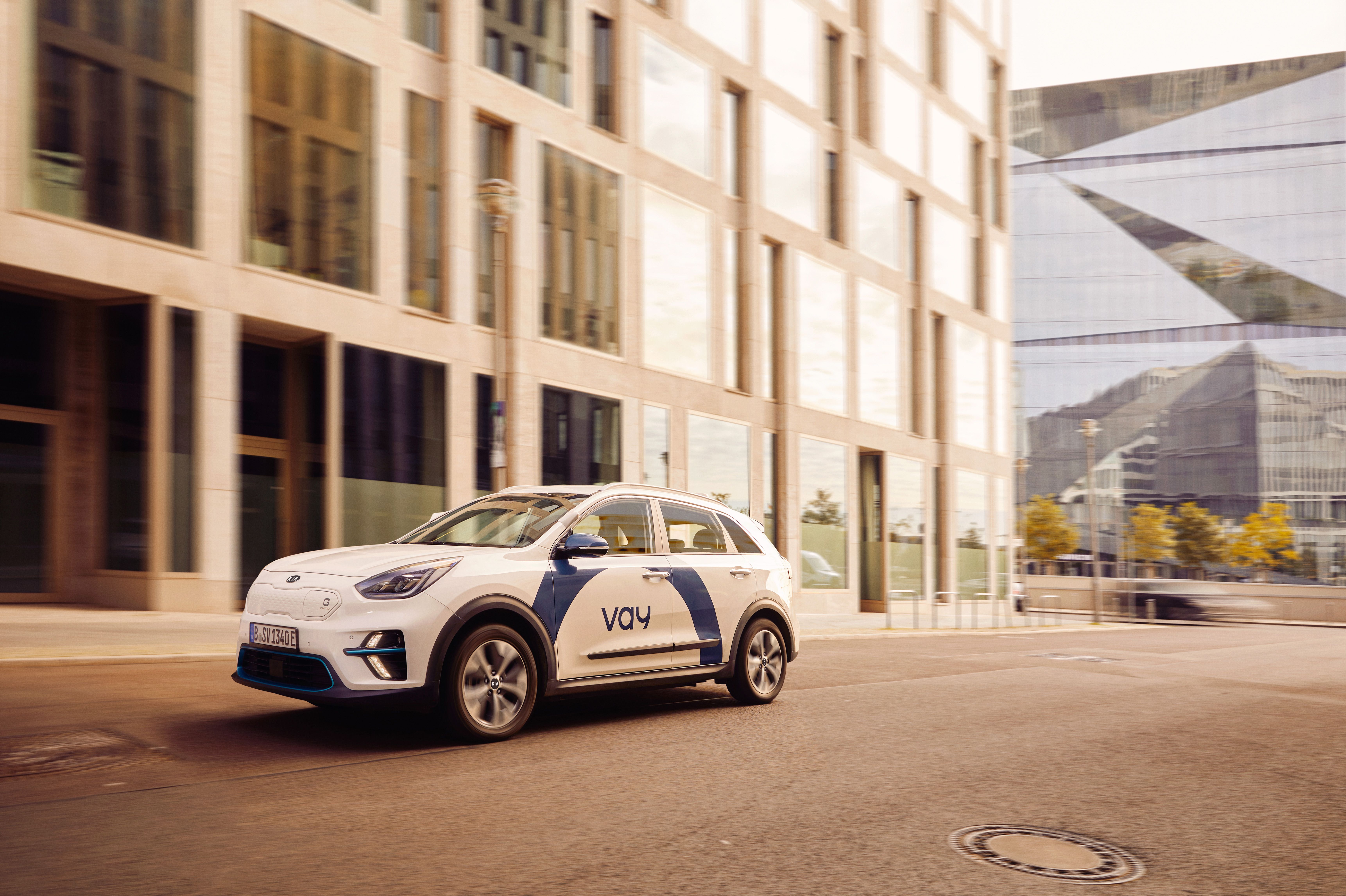
At Vay, we deeply believe that there is a better way to optimize utilization rate of car sharing and ride hailing services. We are building a unique mobility service which will challenge private car ownership and introduce a new way of moving in big urban environments. The underlying technology empowering this new approach is teleoperation (remote vehicle control). Vay will be the first company launching a mobility service which is capable of delivering empty vehicles to our customers at the desired pick up location and taking them over once the trip is over. Our vehicle fleet is remotely controlled by remote drivers (teledrivers) who can be at the same city, different city or even in a different country compared to our customers.
As you might imagine, one of our core modalities is ultra-low latency video streaming. In order to be able to safely control what essentially is a 1.5 ton robot at higher speeds on public streets without a safety driver, we need to be able to achieve glass-to-glass video latency below 200ms.
What is glass-to-glass video latency?
Glass-to-glass latency refers to the time duration of the entire chain of the video pipeline. From the moment a light source hits the CMOS sensor on the camera mounted on the vehicle to the final rendering of the image on the computer screen of the teledriver. All components in this chain (camera frame capture, ISP post-processing, encoding, network transmission, decoding, screen rendering) add a certain amount of delay to the feedback for the teledriver.
Depending how vertically integrated you are or how good instrumentation of the video pipeline you have, you might be forced to look at your entire system as a blackbox in order to measure with high accuracy glass-to-glass latency and include all contributors which affect the final latency number.
How not to measure glass-to-glass video latency
Like with any other measurement methodologies, there are more and less precise ways to do this. The usual method that many use is putting a high precision digital stopwatch in front of the camera and capturing a photo while the stopwatch is being rendered on the screen. This is quite a manual effort with low precision (due to the refresh rate of the stopwatch screen) and does not provide you with a good understanding of the glass-to-glass latency value distribution over time. Some other less scientific methods involve snapping your fingers in front of the camera and looking at the rendered image on the screen in order to have a subjective feeling on the latency of the system.
How to do it properly?
At Vay, we recognized quite early in the project the importance of having the high precision and reliable tool for measuring glass-to-glass latency. The glass-to-glass latency measuring tool (which is based on this paper) became important in evaluating and comparing different camera models and hardware compute platforms. Given that we found it quite useful in our context, we decided to open source it. We hope you will find it useful as well for understanding your end-to-end system performance and consider it over more expensive alternatives.
Basic principle behind the tool is to centralize the emitting of the light source (LED) on the camera lens and its detection (phototransistor) on the computer screen. The centralization approach eliminates the need for time synchronization which increases the precision of this method. Once that is in place, we are able to calculate the time delta between when the light source is activated (LED triggering is usually measured in microseconds) and when it is detected. Another benefit of this approach is that you are able to execute multiple measurements over time in order to get the latency distribution over time.
In our repo, you will find an affordable setup which is based on Arduino Uno and related sensors (LED and phototransistor). By running a simple Python script, a measurement test will be kicked off for a predefined number of cycles. The end result is a chart that shows the glass-to-glass video latency distribution.
Btw, we are hiring!
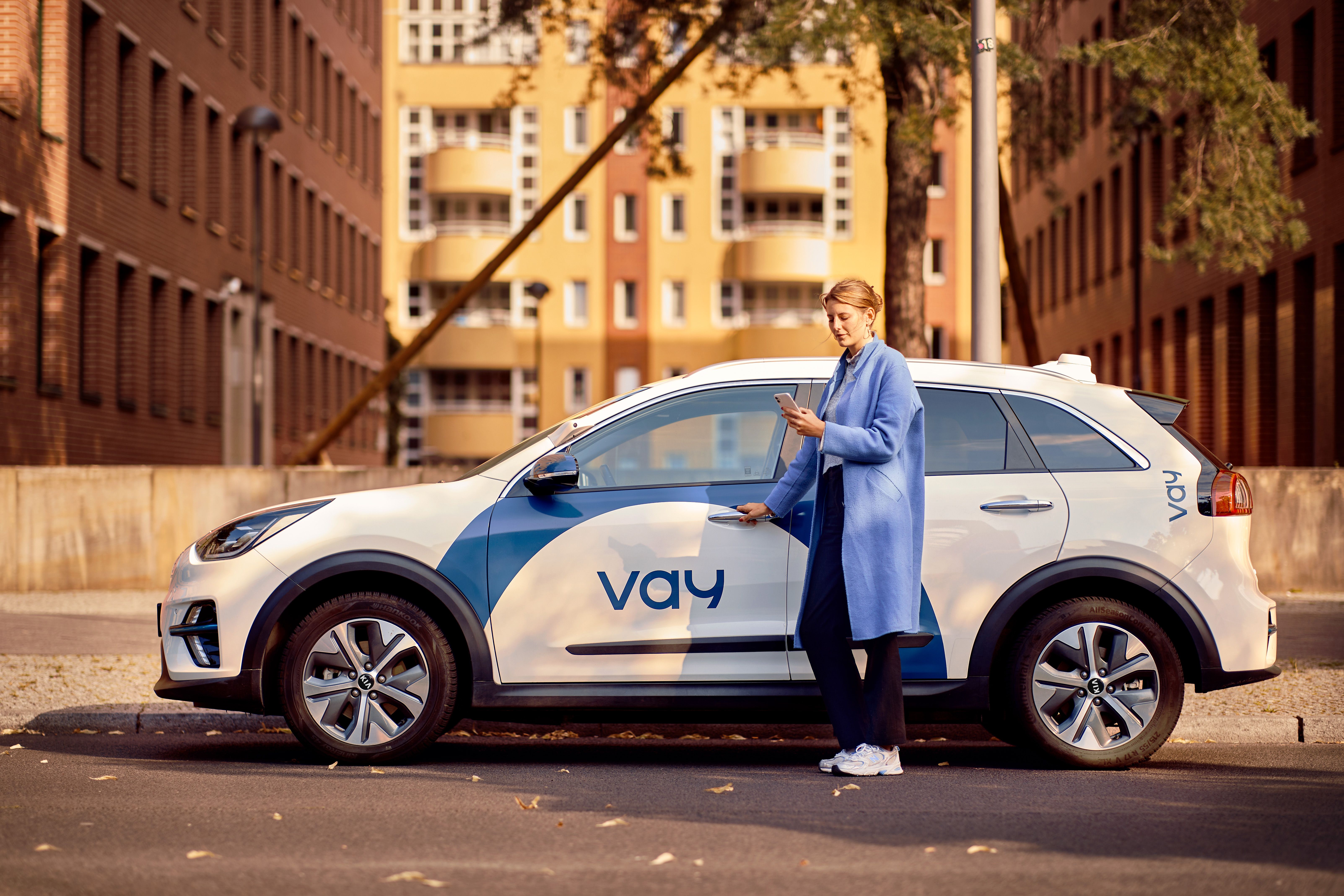
In case you are excited about working on a deep tech project which includes several engineering disciplines (robotics, perception, video, control, data science embedded, cloud, mobile app development, etc.) and would like to contribute to Vay’s new mobility service concept, do check out Vay’s Career page. We are Berlin based but we are offering remote work options across different roles.